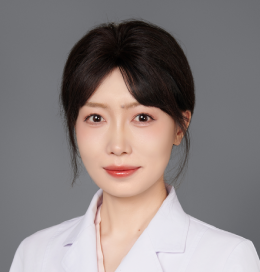
Plastic and Aesthetic Surgeon, Peking Union Medical College Hospital Postdoctoral Fellow in Peking Union Medical College Hospital Member of the Facial Rejuvenation Group, Society of Plastic Surgery, Chinese Medical Association Member of the Perineal Plastic Surgery Group, Society of Plastic Surgery, Chinese Medical Association Research Focus: Development of an AI-based diagnosis and treatment system for pathological scars AI-driven quantitative analysis and 3D visualization for perioperative breast plastic surgery Published 9 SCI papers as first/co-first author.
Abstract submitted for the 5th International Keloid Symposium
TITLE: Establishment of a Deep Learning-Based Automated Segmentation and Blood Perfusion Prediction System for Keloids**
AUTHORS: Shuo Li, Mengdi Zhang, Binghan Wang
AUTHORS ‘AFFILIATIONS: Department of Plastic Surgery, Peking Union Medical College Hospital, Chinese Academy of Medical Sciences and Peking Union Medical College,
Beijing, 100730, People’s Republic of China
BACKGROUND: Keloids are pathological scars commonly arising after skin trauma, mediated by various pathological mechanisms. They exhibit dynamic changes, high internal heterogeneity, and biological characteristics similar to malignant tumors, with high recurrence rates and prolonged treatment cycles. Blood perfusion maps based on Laser Speckle Contrast Imaging (LSCI) can effectively assess the blood perfusion and growth status of keloids. However, conventional LSCI-based blood flow measurement processes are time-consuming and labor-intensive. Therefore, this study aims to develop a deep learning-based automated segmentation and blood perfusion prediction system for keloids, enabling remote, efficient, and objective evaluation of their blood perfusion and growth status.
METHODS:This study retrospectively and prospectively included 3,000 patients, collecting macroscopic photographs of keloids and LSCI-measured blood perfusion maps. Using deep learning, macroscopic photographs were utilized as input to construct an automated keloid segmentation model and an image-based blood perfusion prediction model. During model development, robustness was validated by altering imaging conditions (e.g., shooting angles) or adding noise to images (e.g., brightness, contrast, and blur adjustments). In the prospective cohort, the constructed models were used to evaluate blood perfusion values before and after keloid treatment, and the results were compared with measured values to verify the accuracy of the models in real-world follow-up scenarios.
RESULTS:In the retrospective study, the automated segmentation model achieved a correlation of 0.96 with measured values in identifying keloid area size. The image-based blood perfusion prediction model demonstrated a correlation of 0.75 between predicted and measured values. The blood perfusion prediction model exhibited superior robustness against noise interference compared to traditional models. In the prospective cohort study, the blood perfusion prediction model achieved correlations of 0.64 and 0.76 with measured values before and after treatment, respectively.
CONCLUSIONS:By integrating multi-stage deep learning models, this study established an automated segmentation and blood perfusion prediction system for keloids based on macroscopic photographs. The system demonstrated high segmentation accuracy and blood perfusion prediction performance, enabling objective, remote, and precise assessment of keloid growth status and severity. It facilitates early identification of poor prognosis cases, personalized follow-up monitoring, and guidance for secondary intervention timing. This approach significantly enhances clinical diagnosis and treatment outcomes, providing robust support for refined and personalized management of keloid patients.